Analytical Workflow Management and Your Data Strategy
A robust analytical data management platform is essential for elevating your lab's productivity
Analytical data is the core of all chemical research. Whether you are developing small molecule pharmaceuticals or testing materials used in consumer products, you rely on analytical data to verify the identity and quality of chemicals. This is why work is typically structured around efficiently getting the necessary data.
Unfortunately, inadequate analytical data management can slow down chemistry workflows. Scientists must spend considerable time converting file types or searching for missing results. Most experimental data also lack the necessary formatting or metadata to be reusable or interoperable. For instance, if you are synthesizing a molecule that follows the same synthetic steps you have used in the past, you should be able to leverage previous data without repeating your work. The inability to leverage past data leaves scientists having to unnecessarily repeat experiments and creates challenges in implementing labor-saving programs, such as machine learning.
This article explains why poor chemical data strategy compromises efficiency and explores how implementing a chemically intelligent, vendor-neutral analytical data management platform can increase productivity.
Why do you need a data strategy?
Raw data is not valuable in and of itself. It cannot be mined for insight or fed into a machine-learning model if it lacks the necessary contextual information or has not been adequately processed. In fact, low-quality results often reduce efficiency since scientists must spend their time cleaning up dirty data, converting file formats, or searching for relevant results.
Are more context, standardization, and metadata the key to useful data? Unfortunately, too much structure can also be a barrier to productivity. Experimental chemists don’t want to spend time filling out metadata, particularly if it doesn’t meaningfully contribute to success. Companies must develop data strategies that align with overall business objectives and then implement tools that help achieve that goal.
While many technologies play a role in realizing an effective data strategy, a data management platform designed for analytical data is essential. It acts as the backbone that connects the entire system.
Analytical workflows and the data diversity dilemma
Modern analytical chemical research involves many analytical methods. When elucidating the structure of an unknown impurity, for example, an analytical chemist will typically use nuclear magnetic resonance (NMR) and liquid chromatography-mass spectrometry (LC-MS) for an initial assessment, possibly followed by a combination of gas chromatography, MS, infrared spectroscopy, and other techniques. Each of these experiments uses a separate instrument, meaning a different type of file, which is often stored in a different location.
While this data diversity is necessary for the analytical scientist to do their work, it can also lead to many issues, such as:
- Analytical chemists must review their data by cross-referencing between multiple applications, which is inefficient.
- Team members will struggle to find these results when completing regulatory documentation because they don’t know where the files are located or they lack contextual information. The experiments must be repeated if they cannot find the correct files.
- Data scientists cannot use experimental data because it is not in a standardized, machine-readable format.
One approach to reducing data diversity is to use instruments from a single vendor. However, replacing functional equipment to homogenize the generated data is financially irresponsible. Moreover, chemists want the freedom to choose the right tools for their specific work—there are many examples of equipment being better suited for a niche area of chemistry.
While many technologies play a role in realizing an effective data strategy, a data management platform designed for analytical data is essential. It acts as the backbone that connects the entire system.
Ultimately, diversity of data is unavoidable, so organizations should adopt systems that support this reality.
Improving efficiency with chemical data platforms
What would an analytical data platform that embraces data diversity look like?
First, the platform must be compatible with many types of analytical methods. It’s convenient for scientists to access all their analytical data in a common interface to compare results and make better decisions easily. This also improves efficiency since users do not need to spend time switching between multiple programs or converting files.
The scientific data platform should also be vendor neutral. Most research teams use analytical instruments made by several equipment manufacturers, which often means many software packages to process that data. It is challenging to compare or consolidate results, and scientists must be trained to use many applications.
An analytical data platform should also be chemically intelligent. Chemical intelligence means understanding chemical structures, sub-structures, nomenclature, spectral and chromatographic parameters (e.g., peaks, retention time), and formulas. One popular tool for aggregating chemical data is Excel; however, this application cannot “read” a chemical structure, which means it is usually impossible to search by chemical structure or name. Using a platform with chemically intelligent search and data visualization options helps scientists save time when looking for data and reduces the need to repeat experiments.
Finally, analytical data should also be shared with contextual information. A chromatogram has limited uses —you need to know which material was analyzed and the experimental conditions used. However, manually inputting this information is time-consuming and tedious. An analytical data management platform that automates data marshaling and reduces the burden on scientists will help organizations create efficient workflows while removing the data management burden from scientists.
Case studies in analytical data workflow management
How does an analytical data management platform operate in practice, and how does it affect the workflow of scientists? Here are some examples:
Forced degradation: Forced degradation testing involves many experiments and many types of analytical data. A biopharmaceutical company wanted a tool to help them gather all their data together and compare results between experiments. Using an analytical management tool, the company has been able to consolidate its forced degradation data into a single dashboard. This includes indicators to help users see which tasks are complete, in progress, or have yet to begin.
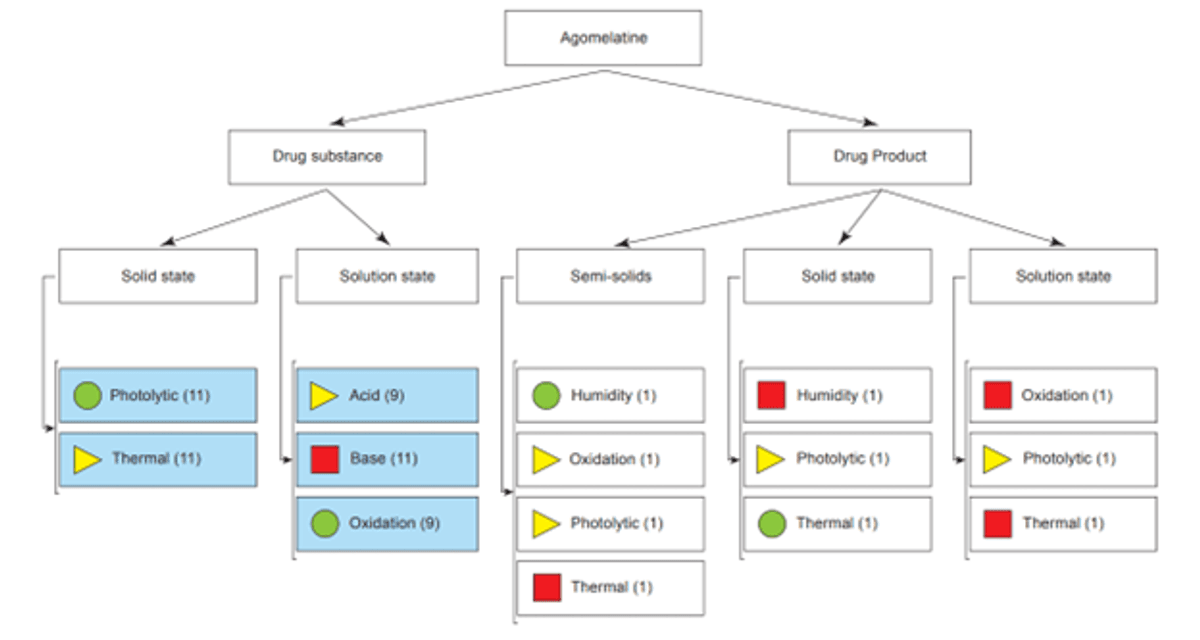
Automating structure verification: Structure verification requires a significant amount of routine work, where each analytical result is processed, analyzed, and then connected to a structure. While this work is necessary, it is also time-consuming. Another biopharmaceutical company implemented an automated structure verification system based on centralized management of NMR and LC/MS data, allowing their analytical chemists to focus on elucidating novel structures.
Democratizing & streamlining high-throughput experimentation: High-throughput experimentation (HTE) in pharmaceutical discovery has been experiencing a renaissance thanks to advancements in robotics and instrumentation. However, the analytical results are often disconnected from the synthetic information, requiring manual data interpretation and connection of analytical results to experiments. This is challenging for a 96-well plate because the scientist must often manually associate results to each well. Analytical results are heterogeneous and lack the necessary context, meaning the data requires engineering for machine learning applications. By streamlining HTE workflows in a software platform, more scientists can use high-throughput methodologies and make effective decisions quickly with analytical results connected to experiment wells. Meanwhile, data scientists have the homogenized contextual data they need to develop robust models.
Implementing your data management strategy
Of course, your data management strategy isn’t just about your technology. Humans are ultimately responsible for operating these systems, and even world-class scientists can resist change. Transformation is most successful when all stakeholders benefit and there is a well-developed strategy for data use—whether immediately or in the future.
Updating and implementing your data strategy can be a win for everyone. Researchers don’t want to spend their time fighting with spreadsheets, chasing down analytical results, or completing basic NMR processing. Implementing the right analytical data management platform will accelerate workflows to build efficiency and productivity for R&D organizations while allowing scientists to focus on their science.