Why You Need a Lab Integration System
Integrated data streams are vital to making data-driven decisions
Every lab manager wants to make data-driven decisions, but effective data management is challenging. All too often, data originating from various lab assets and informatics platforms are siloed apart, rendering it nigh impossible to make holistic decisions accounting for all available datapoints. This is where lab integration systems can shine.
Associate editor Holden Galusha spoke with Nathan Clark, founder and CEO of Ganymede, about how a lab integration system can benefit your lab, what is needed to implement such a system, and more.
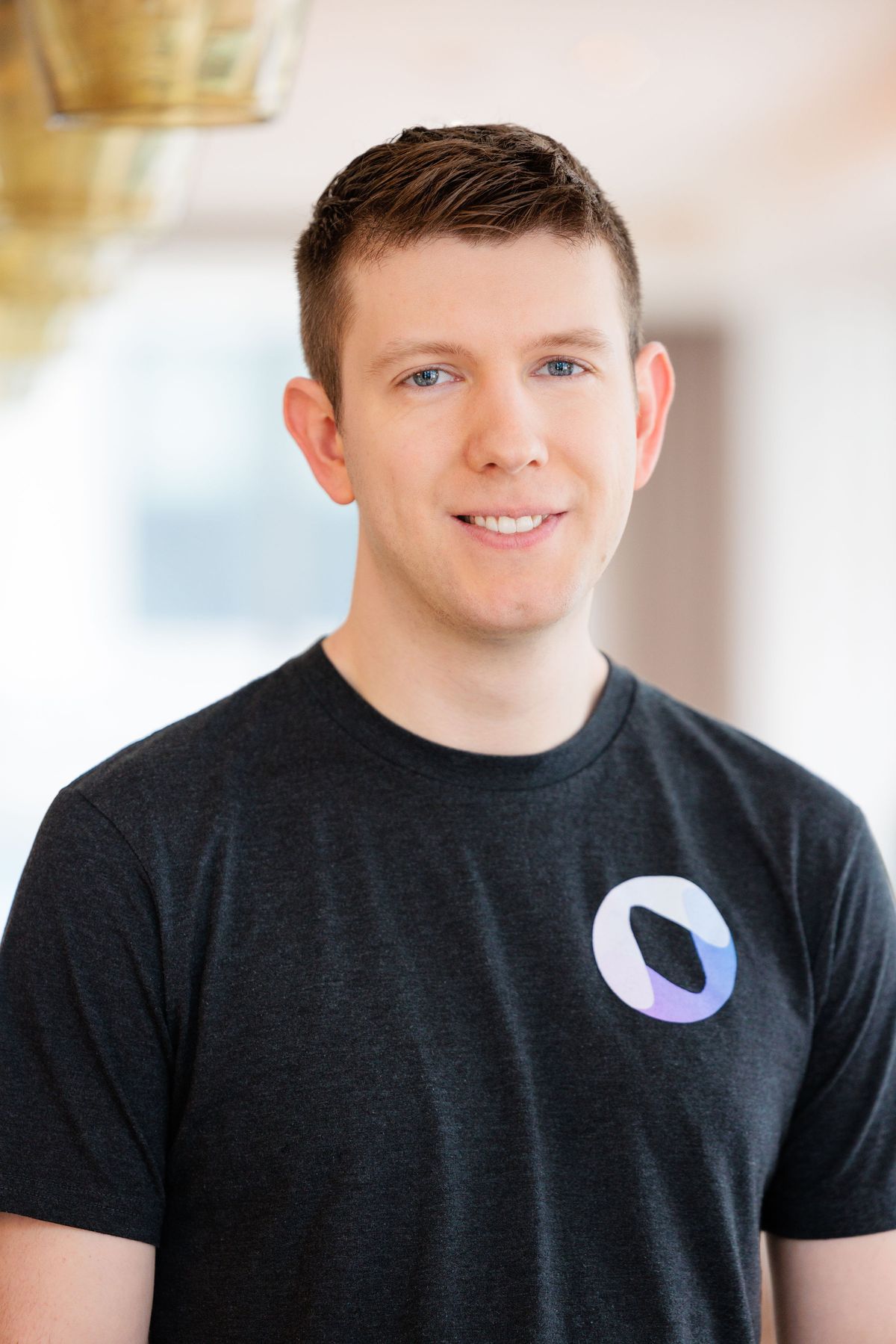
Q: What is a lab integration system, and why is it important? What problems does it solve?
A: All too often, data in labs is trapped in silos. Dozens of different instruments produce data files, which end up stuck on individual lab PCs or USB keys in all kinds of different formats. This scattershot approach to data creates huge issues in terms of busywork for scientists and for data quality. In fact, scientists spend a significant amount of their time on data-related tasks, doing things like moving files manually with USB sticks and manually transcribing data from files or analysis outputs into ELNs, LIMS, and more. Not only is that an unfortunate waste of their time, but it also introduces opportunities for human error.
A lab integration system captures and harmonizes all of a lab’s data. It’s platform-as-a-service software that gathers data from every source into a single cloud-based location. It makes a lab’s data FAIR—findable, accessible, interoperable, and reproducible—and can automatically send that data wherever it needs to go. In short, a lab integration system “desilos” data.
This type of system fixes a huge number of problems, both in terms of time management and data quality. By getting all the data in one place, most of the manual, hands-on work associated with data management is automated away. Scientists get their time back—as much as an entire day a week, in our estimate. Automating data flows also reduces errors. For example, my company’s software can reduce errors by as much as 90 percent.
Q: What requirements are there? What does the lab already need to have to gain max value from an integration system
A: To get the most out of an integration system, labs certainly need some amount of process and infrastructure in place—and, of course, messy data. They need to have enough process stability to be able to lock in automation to some extent, such as established assays. They also should have a built-out wet lab and a suite of instruments that are most likely underutilized. The lab should also have a minimum amount of lab-standard software in place, such as a cloud-based ELN, or cloud infrastructure.
Q: What are the key features of a lab integration system?
A: Because of the complexity associated with wet lab data, we encourage labs to look for features that maximize automation without sacrificing context and metadata. Lab managers should look not only for a number of different technical features, but also for flexibility.
For example, your system should meet your lab’s data wherever it is. It should be able to acquire files off PCs automatically, listen to APIs on instruments, provide forms for users to manually upload files, integrate existing clouds, and listen to SFTP servers for CROs sending data. These files shouldn’t be locked into a black box—you should easily be able to see and sort all the data you have.
Any system should also parse and harmonize data, putting it in an instrument-agnostic format that is FAIR-compliant and that includes metadata. Context and metadata cannot go by the wayside in this process; these elements must be part of the data format, too. That data should be automatically added to a database or data lake that everyone in the lab has access to.
Additionally, your data integration system should have capabilities to reshape or analyze data automatically, so it’s ready for putting into systems of record, and then actually inject that data into some of your lab’s backbone tools, such as ELNs or batch records.
Q: How can a lab integration system help lab managers make data-driven business decisions?
A: I like to say that siloed data leads to siloed thinking. How can labs make meaningful discoveries, generate reproducible experiments, or make big-picture business decisions when information is hidden in different nooks and crannies? Or when context and metadata is lost as soon as a scientist moves onto a different job or position?
A data integration system can help lab managers make decisions on multiple fronts, like comparing across experiments, accelerating drug discovery and program progress tracking, finding novel relationships in R&D, and unlocking process bottlenecks. It also helps lab managers with instrument utilization and efficiency, so they can get ahead of maintaining or replacing instruments in capital planning.
Q: What internal support should the lab have to make best use of the integration system? Would it need internal IT support to get best value from the system?
A: Generally speaking, a data integration system requires some level of IT support, or, at the very least, a data management champion within the organization. Also, it’s best to have an IT professional or a software/data engineer manage the system. This isn’t strictly about technical ability, but rather about having a leader in place to champion the shift in mindset needed for data automation, understand what scientists need, and interpret those needs into the platform.
The truth is that automation and data integration isn’t simple. It’s not just about finding and buying a tech platform with lots of fancy features. Even with solutions designed to minimize building from scratch on AWS, Azure, or GCP, embracing automation requires new thinking about how to standardize business and scientific processes. There’s a cultural element that requires new thinking about data. It’s a significant investment both in terms of IT and business time.
Q: A common concern is that certain workflows will be too complex for low-/no-code platforms. How can a lab manager determine if a low-code solution will accommodate their processes or if they should hire a developer to produce a custom solution?
A: This concern is definitely a real one. Much of science, especially in the wet lab, is too complex for no-code tools and sometimes even low-code solutions. That’s why it’s critical that any integration system allows for in-house developers at life science organizations to also write their own code. It’s also why I recommend that labs always have at least one person on staff who can code, even if just to advise the development of workflows.
Q: How should lab managers approach testing a low-code platform before rolling it out to production in the lab? Is there a way to implement the system in one small portion of the lab as a demonstration before committing to a full implementation?
A: Give it a test drive. It’s always best to start with one assay from a few different instruments—usually the ones that take scientists the most hands-on time—and then build outward. Doing an experiment like this helps demonstrate value and test things in a more agile way. While many older, legacy vendors would advocate for a broad digital transformation that integrates everything at once, I’ve found that tackling too much at once often over-indexes on capturing data and doesn’t focus enough on addressing scientists’ day-to-day problems.
Q: Some lab managers may feel wary putting so much information/reliance on one third-party platform. How would you respond to that?
A: It doesn’t make any more sense these days for scientists to maintain their own servers than it does for doctors to build their own ambulances. In this analogy, keeping all your lab data on paper or local PC files is like using a rickety, homemade go-kart instead of hiring a professional ambulance, just because you can assemble the go-kart yourself.
In fact, a homegrown, on-prem system can be a bigger risk than a third-party solution. Modern cloud platforms like AWS and GCP and Azure are very rigorous about security defaults, so cloud-based SaaS platforms have generally become more technically secure than on-prem solutions. Social engineering is the main risk for companies these days, and your SaaS provider will be far better guarded against this than your company. The truth is that many labs already are in the cloud, at least in part, with ELNs like Benchling or infrastructure providers like AWS. It’s inevitable that biotech will move fully into the cloud in the coming years.
Q: Is the implementation reversible? If it doesn’t serve the lab well, can it easily be removed, or is this a permanent decision?
A: The best implementations are reversible, especially because the best implementations know they’ll need to evolve over time to be successful. Be suspicious of solutions that promise the world right out of the box with no changes. Science changes too quickly for software to stay stagnant.
Q: What does the future of lab integration platforms look like?
A: In the future, we’ll see data integration and automation come to all corners of the lab, including manual assays, which have been largely neglected to date. Organizations will view lab data integration as less of a one-off investment and more like a continuous development process to capture the agile, ever-changing nature of science. We’ll see a move away from closed, proprietary instruments toward developer-friendly platforms, open source, and open data.
Nathan Clark is the founder and CEO of Ganymede, the modern data platform and cloud infrastructure for science. Prior to Ganymede, Nathan was product manager for several of Benchling's data products, including the Insights BI tool and Machine Learning team. Before that, Nathan has a background in machine learning and data systems across financial technology and general technology.