Charting a Course for Implementing Laboratory Automation
Considering where and how to introduce automation in the lab, along with the future of automation
Laboratory automation is beneficial to many labs, but navigating the decisions that come with introducing automation is a challenge. Lab managers must know not only what to automate, but how to build a business case for automation, where to begin automating, and how to ensure a smooth training process.
Associate editor Holden Galusha speaks with Meghav Verma, product manager at the National Center for Advancing Translational Sciences (NCATS/NIH), on what lab leadership should consider when automating lab processes, avoiding the trap of overautomation, and the future of lab automation.
Note: These responses have been edited for clarity and style.
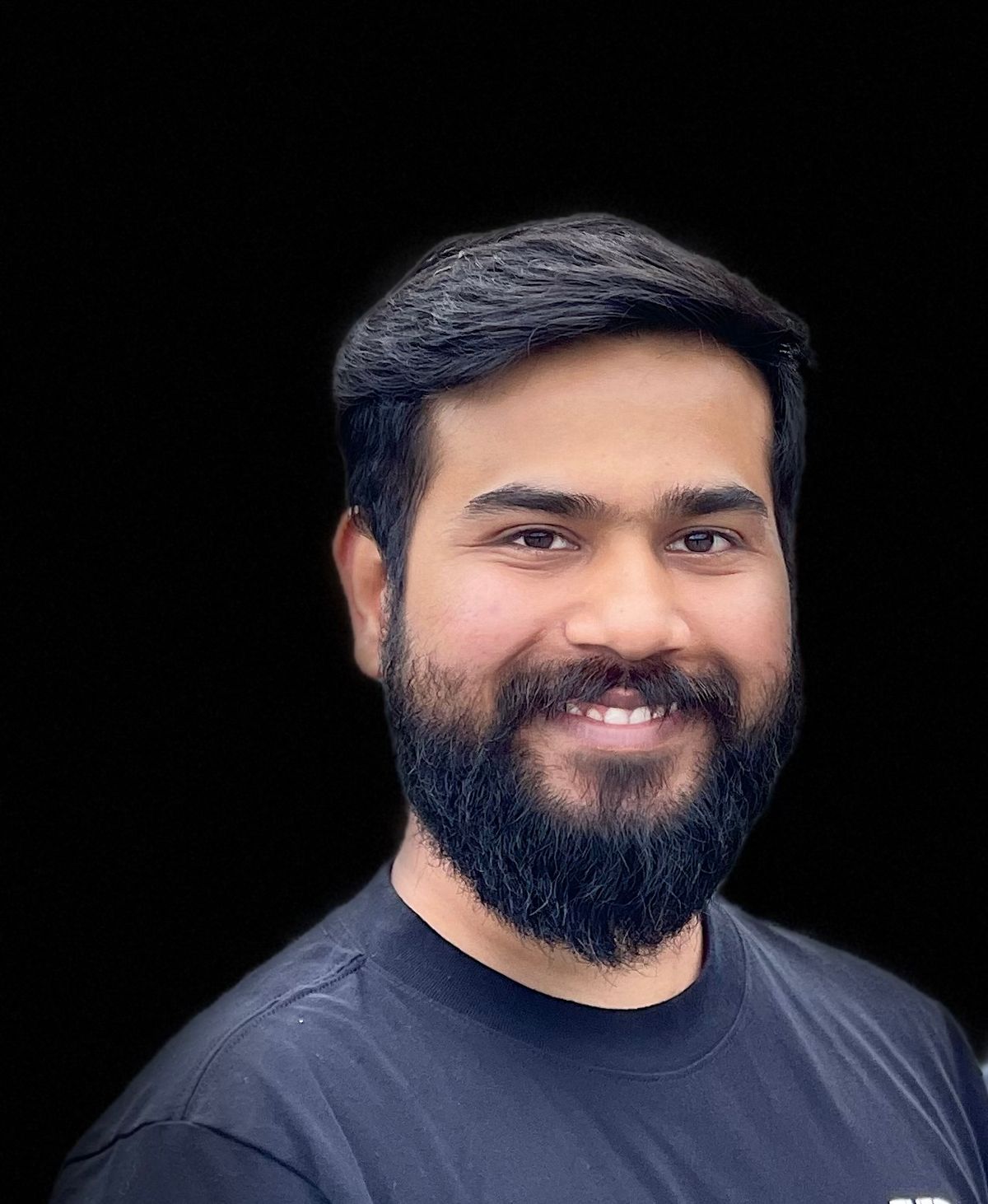
Q: If lab leadership decides to introduce automation, how should they decide which process(es) to automate first?
A: Before deciding which process to automate, there are two things we first must understand: (1) performing an analysis on where the bottleneck lies in the entire process and (2) making sure the current workflow is properly organized and standardized. Without organization and standardization, you can never achieve repeatable results, with or without automation so it is extremely crucial to consider these factors. There are repetitive tasks that might be performed manually by a scientist. For example, in the lab where I’m a contractor at, we are trying to solve the evaporation bottleneck, where we are evaporating a solvent in a microwave vial and capturing the weight of the product before and after the evaporation. This process is quick and simple to execute when it comes to one to two vials, but it becomes an encumbrance when there is a large batch of vials to process, which creates a bottleneck in the workflow. This process can be a clear candidate for automation, visibly benefiting the turnaround time and improving efficiency, which can be tracked to validate the decision of automating this process.
Q: Some benefits of automation cannot be easily quantified, such as decreased risk of repetitive strain injury associated with performing tasks manually. How should a lab manager incorporate such factors into their argument when advocating for automation?
A: With tasks being performed manually, there is always a risk of strain and injury, although it might not be easily quantified. But with manual tasks, there are other problems arising as well. The biggest issue is with repeatability and consistency, coupled with the problem of low throughput. We need to approach the problem in a way where we are able to use hard facts and numbers to show improvement in the process by automating tasks. This method of approaching the issue allows stakeholders to clearly see the benefits of automation in terms of cost and time reduction, which is more likely to gain acceptance and, in turn, also eliminate the risk of strain- and injury-related problems.
Q: The introduction of automated lab equipment moves costs associated with the tasks from operational expenditure to capital expenditure. What are the benefits or downsides of this transfer, if any? How should leadership reframe their view on the ROI needed to justify the cost of automation?
A: With any such decision, we always have to evaluate the short-term and the long-term gains and losses. With purchasing and installing new automation equipment, we always have the upfront cost associated with it, but when comparing the operational costs versus the capital costs over time, the cost of automation comes out to be cheaper. However, this is not a given, and we always have to go through the evaluation process of understanding the long-term gains. We also have to consider the time and opportunity cost that is saved when employing automated solutions. These costs add up in the long run and become a large source of inefficiency in operations.
Q: Successful automation can be seen as a three-way partnership between hardware, software, and training. How can lab managers make that partnership work?
A: I think adoption of automation is a real issue being faced by lab managers in their labs. Oftentimes researchers are accustomed to certain practices which they are confident in, and they do tend to become wary of new processes or equipment to perform the same tasks they were performing earlier. That being said, the onus lies on the lab manager to make sure that the hardware and software is not something that is completely changing the way the scientists were performing their tasks. The selection and implementation of equipment should feel familiar to the user; this will increase the rate of adoption of that technology in the lab. Software should be very simple and intuitive to use so it has the lowest barrier of entry for the scientist to start using it. Trust is an important aspect of this, too. Users need to build trust in the process by verifying the data. All that said, there have to be consistent training programs associated with new equipment to allow the users some time to learn and get used to the system, rather than feeling like being thrown into the deep end of the pool without any experience.
Q: In the past, some companies have reported “overautomating” their processes, such as Tesla’s infamous Model 3 assembly line. In cases such as these, the automation was too ambitious and actually hindered productivity. What are symptoms of overautomation that laboratory managers should look out for? How can leaders be sure that they won’t be overautomating?
A: There’s a saying, “What’s not broken shouldn’t be fixed”. While it should be encouraged to take advantage of the ever-growing and evolving technology in the field of automation, we also have to make sure that just because a technology is new, fancy, and available, doesn't mean that it has a place in your existing workflows in the lab. Every decision of implementing new technology has to be based on a thorough analysis of the requirement for that technology. As long as the decision is based on merit, we can avoid overengineering any process.
Q: What does the future of artificial intelligence and machine learning in laboratory automation look like? What are some ways it’s being used in labs today?
A: There is a plethora of data available in the labs in the form of electronic notebooks. This data consists of parameters and methods used to achieve a certain reaction condition or a product. These notebooks can be relied upon to recreate the same reaction conditions and generate repeatable results. There is a huge opportunity in using this data to automate a lot of the workflows in the labs and make devices more intelligent. One such way is the development of a computational platform, which is being developed in our labs. The idea is to ingest reaction data into a knowledge base and apply machine learning algorithms on it to create a set of reaction conditions/parameters for the robot to run. This creates different methods and protocols for the user to try to generate even more data.
Q: What do you think the next “big breakthrough” in lab automation is?
A: In my opinion, completely autonomous labs are going to be revolutionary. We are still a few years away from achieving a completely autonomous drug discovery cycle, but that would be the next big thing. Making machines perform physical research based on a think tank of actual scientists in the form of data they provide as an input, but with minimal human intervention, can really speed up the process of drug discovery. We would be able to generate consistent, repeatable data at a much quicker rate than we are right now, allowing for more therapeutics and treatments being available to more people for all diseases.
Meghav Verma is currently working for National Center for Advancing Translational Sciences (NCATS/NIH) as a product manager through Axle Informatics, LLC, where his job is to create the vision and goals for developing a state-of-the-art chemistry automation lab and manage the ASPIRE program. He works with the engineering team to develop various fixed/mobile robotic systems to automate the labs to help the institute perform faster and more efficient research towards drug discovery and therapeutics development for rare diseases. Meghav will be a featured speaker at the Lab Manager Leadership Summit, taking place April 29- May 1, 2024 in Denver, CO. See the full agenda and register here: https://summit.labmanager.com/leadership